Conférencier invité
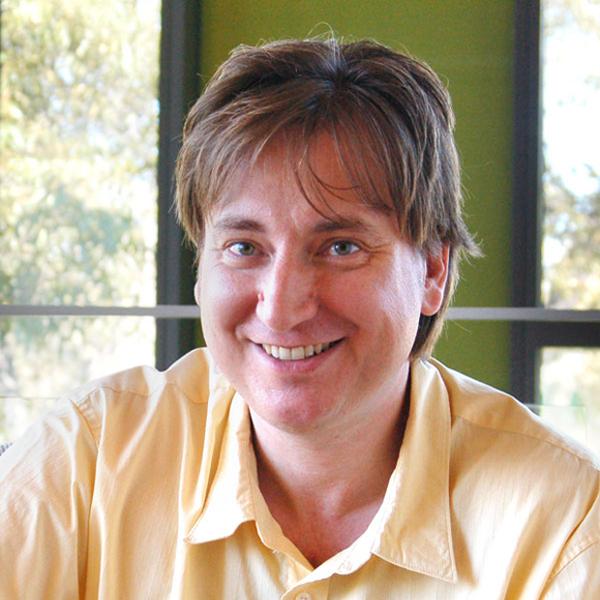
Mathieu Desbrun
Inria Saclay - on leave Caltech
Dimensionality reduction via geometry processing
A common and oft-observed assumption for high-dimensional datasets is that they sample (possibly with added noise) a low-dimensional manifold embedded in a high-dimensional space. In this situation, Non-linear Dimensional Reduction (NLDR) offers to find a low-dimensional embedding of the data (i.e., unfold/unroll the original dataset in R^d for a small value of d) that creates little to no distortion so as to reduce the computational complexity of subsequent data processing. Given the clear geometric premises of this task, we revisit a series of well-known NLDR tools (such as ISOMAP, Locally-Linear Embeddings, etc) from a geometric processing standpoint, and introduce two novel geometric manifold learning methods, Parallel Transport Unfolding (PTU) and Spectral Affine Kernel Embedding (SAKE). These contributions leverage basic geometry processing notions such as parallel transport or generalized barycentric coordinates. We show that tapping into discrete differential geometry significantly improves the robustness of NLDR compared to existing manifold learning algorithms. Somehow surprisingly, we also demonstrate that we can trivially derive from NLDR the first eigen-based shape editing technique, hinting at the generality of our approach.
|